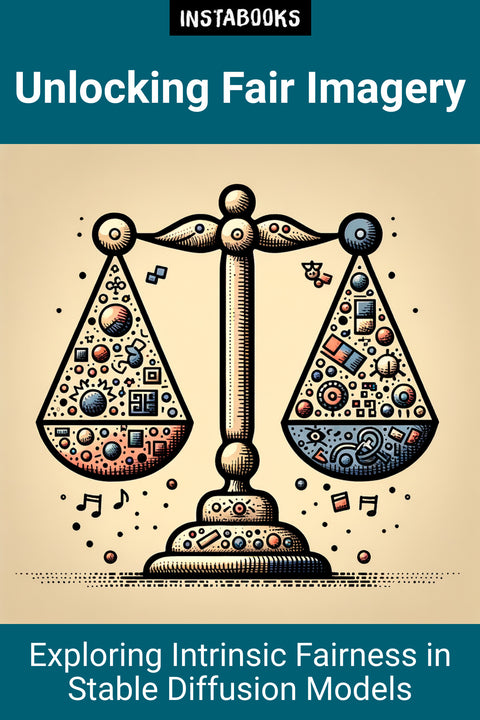
Instabooks AI (AI Author)
Unlocking Fair Imagery
Exploring Intrinsic Fairness in Stable Diffusion Models
Premium AI Book (PDF/ePub) - 200+ pages
Introduction
In the modern landscape of artificial intelligence, "Unlocking Fair Imagery: Exploring Intrinsic Fairness in Stable Diffusion Models" provides a crucial examination of fairness in text-to-image models. These models, capable of generating photo-realistic images, often inadvertently propagate demographic biases, such as racial or gender stereotypes. As a response to this pressing issue, this book embarks on a journey to uncover intrinsic fairness within these models, focusing especially on Stable Diffusion.
Understanding Demographic Biases
Beneath the stunning visuals generated by Stable Diffusion lie biases that mirror societal stereotypes. Our exploration begins by dissecting these biases, revealing how they are embedded within the intricate processes of text-to-image conversions. Through extensive research, the book sheds light on the demographic imbalances present in these models, setting the stage for understanding why fairness is a pivotal concern.
Methodological Approaches and Debiasing Techniques
The journey into fairness continues as we delve into groundbreaking methodological approaches aimed at unlocking intrinsic fairness. By identifying text prompt-diffusion bonds as a bias source, the book introduces novel methods to perturb text conditions. This innovative technique stands apart from traditional training-based debiasing methods, offering a fresh perspective on achieving unbiased image generation while maintaining image-text alignment and quality.
Fairness-Aware Training Protocols
As Stable Diffusion models evolve, so too must our approach to their training. This book emphasizes leveraging the models' intrinsic properties, challenging readers to consider the potential for unbiased outputs without excessive external tuning. By designing experiments that pinpoint and mitigate biases, readers gain insights into complex training protocols that enhance model fairness.
Implications and Future Directions
The implications of achieving fairness in Stable Diffusion extend beyond academia, impacting fields like art, advertising, and education. This book discusses the transformative power of fairer image generation, which can help diffuse stereotypes and foster inclusive representations. Additionally, it covers current developments, such as NeurIPS 2023 workshops, that reinforce the importance of fairness in machine learning.
Conclusion
Comprehensive and timely, "Unlocking Fair Imagery" is an indispensable resource for those seeking to understand and implement fairness in AI models. It not only uncovers the methodologies behind intrinsic fairness but also projects its significant impact on creative and educational domains. Readers will leave with a nuanced understanding of how to harness fairness within Stable Diffusion models.
Table of Contents
1. Demographic Biases in AI- Understanding Biases
- Impact on Image Generation
- Case Studies and Analysis
2. Intrinsic Fairness Explained
- Conceptual Foundations
- Empirical Findings
- Unlocking Fairness
3. Methodological Insights
- Text Prompt Analysis
- Diffusion Process Examination
- Novel Approaches
4. Debiasing Techniques
- Traditional Methods
- Innovative Solutions
- Case Implementation
5. Fairness-Aware Training
- Protocol Design
- Experimentation Strategies
- Achieving Balance
6. Fields of Application
- Artistic Expression
- Advertising Standards
- Educational Impact
7. Recent Developments in AI Fairness
- Notable Workshops
- Papers and Publications
- Community Engagement
8. Implications for Society
- Stereotype Mitigation
- Inclusivity in Media
- Policy Considerations
9. Future Trajectory
- Evolving Techniques
- Long-term Goals
- Ethical Challenges
10. Stable Diffusion in Detail
- Technology Overview
- Model Architecture
- Use Cases and Limitations
11. Designing Fair AI Systems
- Principle Foundations
- System Integration
- Continuous Learning
12. Concluding Thoughts
- Reflection on Fairness
- Key Learnings
- Path Forward
Target Audience
This book is intended for AI researchers, data scientists, and tech enthusiasts interested in understanding and implementing fairness in AI models, particularly those focusing on text-to-image synthesis.
Key Takeaways
- Understand demographic biases in Stable Diffusion models and their impact on image generation.
- Explore novel methodological approaches to unlock intrinsic fairness.
- Learn debiasing techniques distinct from traditional training methods.
- Discover the implications of fair image generation for various fields.
- Stay updated on recent developments and future directions in AI fairness.
How This Book Was Generated
This book is the result of our advanced AI text generator, meticulously crafted to deliver not just information but meaningful insights. By leveraging our AI book generator, cutting-edge models, and real-time research, we ensure each page reflects the most current and reliable knowledge. Our AI processes vast data with unmatched precision, producing over 200 pages of coherent, authoritative content. This isn’t just a collection of facts—it’s a thoughtfully crafted narrative, shaped by our technology, that engages the mind and resonates with the reader, offering a deep, trustworthy exploration of the subject.
Satisfaction Guaranteed: Try It Risk-Free
We invite you to try it out for yourself, backed by our no-questions-asked money-back guarantee. If you're not completely satisfied, we'll refund your purchase—no strings attached.